My research interests include computer vision and machine learning, where I lead a young and energetic research team that has published more than 100 papers in related top peer-review conferences and journals (e.g. CVPR, NeurIPS, TPAMI, TIP etc). I was the founding Chair for IEEE Computational Intelligence Society, Malaysia chapter.
Also currently, I serve as the Associate Editor of Pattern Recognition (Elsevier), and have co-organized several conferences/workshops/tutorials/challenges related to computer vision/machine learning. I was the recipient of Malaysia Scopus Research Excellence Awards (Research Innovations) in 2024, Top Research Scientists Malaysia (TRSM) in 2022, Young Scientists Network Academy of Sciences Malaysia (YSN-ASM) in 2015 and Hitachi Research Fellowship in 2013. Besides that, I am also a senior member (IEEE), Professional Engineer (BEM) and Chartered Engineer (IET).
During 2020-2022, I was seconded to the Ministry of Science, Technology and Innovation (MOSTI) as the Undersecretary for Division of Data Strategic and Foresight.
Highlights:
10/2024: One(1) paper to appear in NeurIPS-2024. Please see Project Page.
10/2024: One(1) paper to appear in EMNLP-2024. Please see Project Page.
02/2024: One(1) paper to appear in CVPR-2024. Please see Project Page.
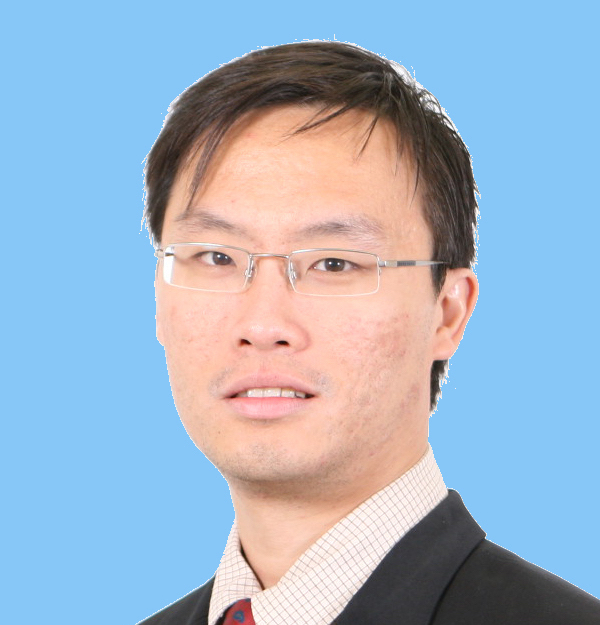
professor at Universiti Malaya
Latest Works
Ferrari: Federated Feature Unlearning via Optimizing Feature Sensitivity Star
H. Gu, W.K. Ong, C.S. Chan and L. Fan
NeurIPS 2024 (acceptance rate: 4043/15671 ~ 25.8%)
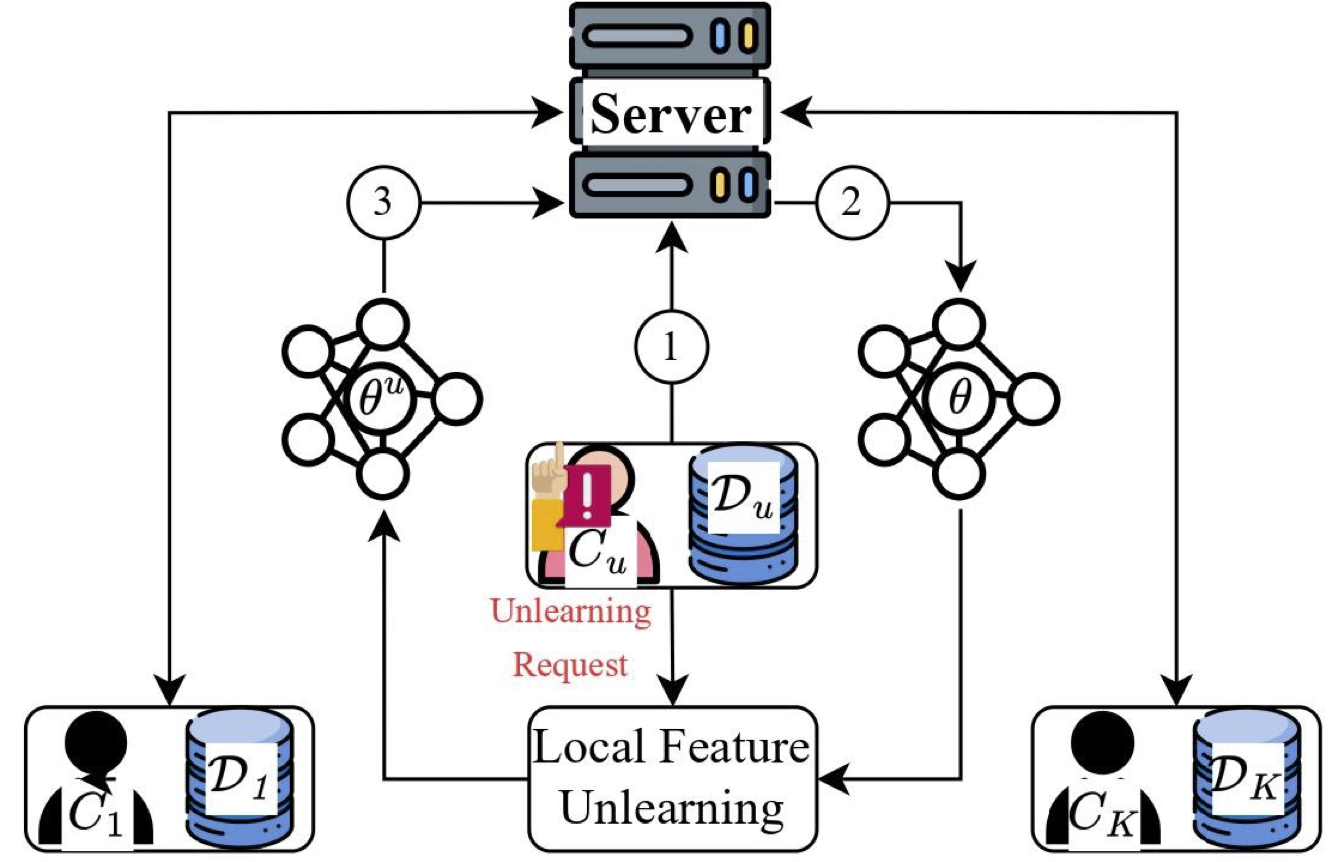
We propose Ferrari, a federated feature unlearning framework that allows a single client to unlearn specific features from a trained global model by locally optimizing feature sensitivity, without requiring participation from other clients.
pdf poster slide codeInteractDiffusion: Interaction-Control for Text-to-Image Diffusion Model Star
J.T. Hoe, X. Jiang, C.S. Chan, Y-P. Tan and W. Hu
CVPR 2024 (acceptance rate: 2719/11532 ~ 23.6%)
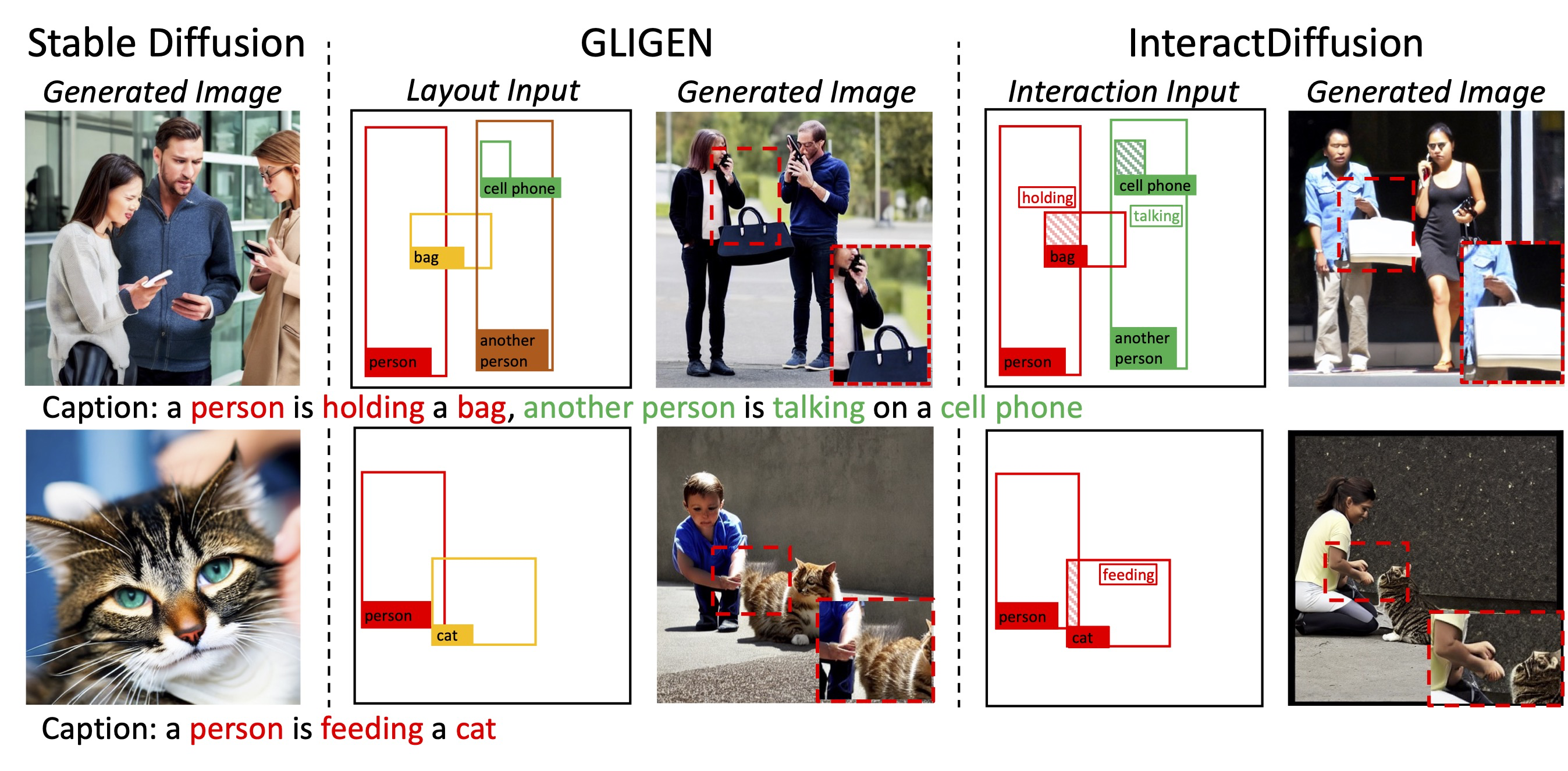
Existing methods lack ability to control the interactions between objects in the generated content. This paper proposes a pluggable interaction control model, called InteractDiffusion that extends existing pre-trained T2I diffusion models to enable them being better conditioned on interactions.
pdf poster code video demoSFAMNet: A scene flow attention-based micro-expression network Star
G-B. Liong, S-T. Liong, C.S. Chan and J. See
Neurocomputing (2024)
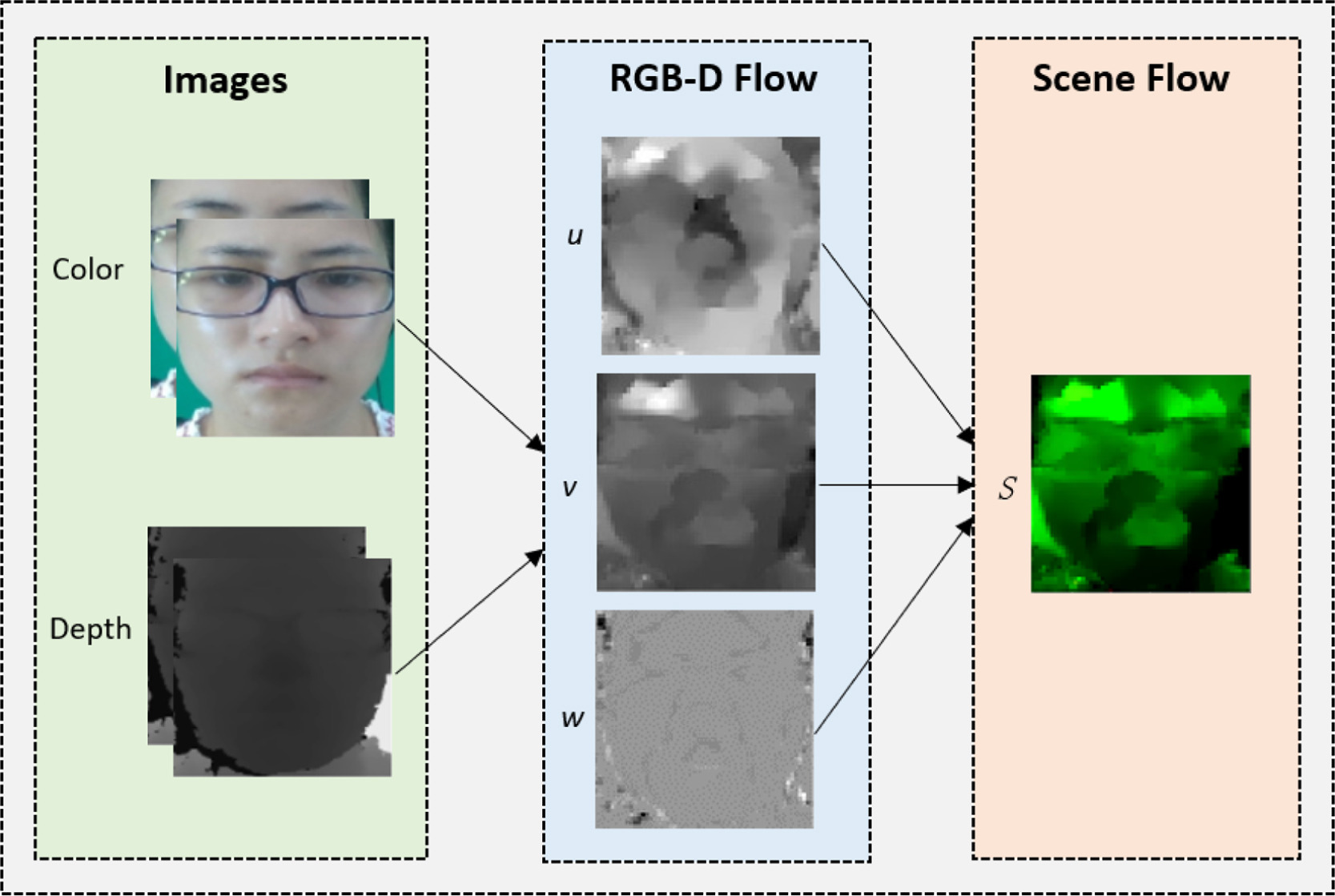
This paper proposes the first Scene Flow Attention-based Micro-expression Network, namely SFAMNet. Extensive experiments performed on three tasks: (i) ME spotting; (ii) ME recognition; and (iii) ME analysis on the multi-modal CAS(ME)^3 dataset indicate that depth is vital in capturing the ME information.
pdf code